Abstract
The strength of quantum correlations is bounded from above by Tsirelson's bound. We establish a connection between this bound and the fact that correlations between two systems cannot increase under local operations, a property known as the data processing inequality (DPI). More specifically, we consider arbitrary convex probabilistic theories. These can be equipped with an entropy measure that naturally generalizes the von Neumann entropy, as shown recently in Short and Wehner (2010 New J. Phys. 12 033023) and Barnum et al (2010 New J. Phys. 12 033024). We prove that if the DPI holds with respect to this generalized entropy measure then the underlying theory necessarily respects Tsirelson's bound. We, moreover, generalize this statement to any entropy measure satisfying certain minimal requirements. A consequence of our result is that not all the entropic relations used for deriving Tsirelson's bound via information causality in Pawlowski et al (2009 Nature 461 1101–4) are necessary.
Export citation and abstract BibTeX RIS
1. Introduction
Quantum mechanics departs fundamentally from any classical theory by allowing non-local correlations [8]. The existence of such correlations has been extensively verified in experiments (up to a few loopholes); see, e.g., [3]. As was shown by Bell, these correlations imply that the world is not both local and realist, two standard assumptions underpinning the classical mechanical worldview [8]. Apart from their fundamental theoretical interest, non-local correlations are also of technological importance, for example as the essential ingredient in Ekert-style quantum cryptographic schemes [15].
However, there is a limit to how much local realism is violated. The strength of quantum correlations are themselves upper bounded by Tsirelson's bound [12, 25]. This is a non-trivial bound, because it can be conceived to violate Bell inequalities more than quantum theory, without having a theory which is signalling (allows instantaneous information transfer across space). For example it is possible to conceive of PR-boxes, also known as non-local boxes, hypothetical systems that maximally violate the CHSH Bell inquality, without being signalling [23].
The question then arises as to whether one can associate a fundamental assumption about nature other than non-signalling with Tsirelson's bound. Such an assumption could then be labelled as a fundamental principle underpinning quantum theory, and could possibly form part of a much-sought set of principles from which quantum theory could be derived.
There has already been significant effort in this direction. For example, it is now known that the existence of maximally Bell-violating correlations would lead to some communication complexity problems becoming trivial [9, 10], the possibility of oblivious transfer [26], weaker uncertainty relations [21], general invalidation of quantum theory locally [5] and severely limited dynamics [7, 16].
A recent string of related papers have, moreover, been concerned with a principle called information causality [2, 11, 22]. A great advantage of this principle is that the exact Tsirelson's bound is recovered, i.e. it rules out any stronger correlations, not just the maximally strong ones. The principle amounts to placing a limit on how well two separated parties can perform in a particular game (van Dam's game [26]) where they share a resource state. This limits the resource state in such a way that Tsirelson's bound is recovered. While the original interpretation of information causality as a particularly simple generalization of non-signalling has been questioned (see, e.g., [24]), the principle is—as mentioned above—powerful.
Intriguingly, in the proof that information causality holds in quantum theory, a specific limited set of information-theoretic theorems are used. One may thus replace information causality as a postulate with those information-theoretic theorems. This is attractive if one seeks an information-theoretic set of principles for quantum theory. In order to discuss the validity of such theorems outside of quantum theory, however, one needs definitions of the relevant entropies for general probabilistic theories. Fortuitously, such definitions were recently proposed and investigated in [4, 19, 24]. In [4, 24], information causality is also discussed. In [4], three sufficient conditions under which a generalized probabilistic theory respects information causality are determined. In [24], it is shown that if one follows the information causality proof in the case of box-world, the theory with PR-boxes and all other non-signalling distributions, the proof breaks down at the point where one needs to assume the so-called strong subadditivity of entropy. An alternative approach to deriving information causality from more basic entropic principles appears in [18]. These recent works when taken together suggest that one may hope for a small and operationally motivated set of information-theoretic relations from which Tsirelson's bound, and perhaps even quantum theory, can be derived.
We here investigate the data processing inequality (DPI) as such a principle. This essentially states that correlations, quantified via conditional entropies, cannot increase under local operations; see figure 1. In order to define this in general, we use an entropy proposed in [24], which naturally generalizes the von Neumann entropy (and reduces to the latter in the case of quantum theory). We prove that, surprisingly, this generalized DPI alone implies Tsirelson's bound.
Figure 1. The data processing inequality states that the correlations between A and B cannot increase under a local operation T on B. More specifically, H(A|B) ⩽ H(A|T(B)).
Download figure:
Standard imageWe proceed as follows. First we describe the framework of generalized probabilistic theories within which we work. Then we define Tsirelson's bound as well as information causality. We go on to describe how to define entropy in an operational manner as in [24]. This is used to define the generalized DPI. We then prove that DPI implies Tsirelson's bound. This involves proving a more general theorem of which the main result is a corollary. Finally, we compare the results to previous ones and discuss the implications and interpretation of the principle.
2. Convex, operational, probabilistic theories
We use the framework of convex probabilistic theories [6, 7, 17]. This amounts to taking the minimalistic pragmatic view that the operational content of a theory is in the predicted statistics of measurement outcomes.
The state of a system by definition determines the probabilities of all possible measurement outcomes. The state is completely specified, again by definition, by the probabilities for the outcomes of k so-called fiducial measurements . k may be significantly smaller than the total number of measurements (e.g. in quantum theory there is a continuum of measurements but k = d2 for a state on a Hilbert space of dimension d). If these fiducial measurements each have l possible outcomes
we will say that the system is of type (k,l).
We can thus write a (normalized) state as a list of P(i|j), denoting the probability of getting outcome i if fiducial measurement j is performed. We represent this by . The normalization of the state is
and is for all valid states independent of the choice of fiducial measurement j. A state is said to be normalized if
and subnormalized if
.
We assume that the set of allowed normalized states is closed and convex (so that any probabilistic mixture of states is an allowed state). We say that a state is pure if it cannot be written as a convex mixture of other states. A theory is defined by the set of allowed states,
, as well as the set of allowed transformations.
Transformations take states to states. They must be linear as probabilistic mixtures of different states must be conserved [7]. Transformations can thus be modelled as , where M is a matrix. If one makes a measurement with several outcomes, each outcome is associated with a certain transform Mi. The unnormalized state associated with the ith outcome is
, and the associated probability of the ith outcome is given by the normalization factor after the transformation:
.
If one is only interested in the probabilities of the different outcomes of a measurement, one can always associate with a transformation {Mi} a set of vectors {Ri} such that . Consequently, for a normalized state
,
is the probability of the ith outcome.
It is also possible to combine single systems to form multipartite systems. If one performs local operations on the systems A and B the final unnormalized state of the joint system does by assumption not depend on the temporal ordering of the operations. A direct consequence of this is the no-signalling principle: measuring system B cannot give information about what transformation was applied to A [7].
We will make the non-trivial but standard assumption that the global state of a bipartite system can be completely determined by specifying joint probabilities of outcomes for fiducial measurements carried out simultaneously on each subsystem. Accordingly, the joint state of two parties is uniquely specified by the list P(ii'|jj'), denoting the probability of getting the outcomes i and i' if one carries out fiducial measurement j on A and j' on B.
For a joint state , the marginal (also called reduced) state of system A, denoted as
, is given by
. Similarly, the conditional marginal state
is defined by

This represents the state of system A after a fiducial measurement l was carried out on system B and the outcome k was obtained.
It was shown in [7] that denoting the vector spaces containing the vectors ,
and
by VAB, VA and VB, respectively, one can relate the spaces by VAB = VA⊗VB (⊗ being the tensor product). One assumes that for
and
we have
. This implies that any
can be written as
with
and
normalized and pure and
[7].
For a transformation on system A defined by the transformation of the joint system is given by
[7]. We demand that transformations MA on any system A are well defined, meaning that
whenever
for all types of system B.
In the following, we will always assume that the set of transformations allowed by the theory includes removing systems (which corresponds to taking the marginal state, as defined above) and adding a system, taking .
We also demand that the theory contains 'classical' systems of type (1,d) for all . We call the trivial classical system of type (1,1) the vacuum (V ). We shall in our proofs, taking inspiration from [6], use the fact that the state of a classical system can be cloned—see lemma 5 in the appendix for the exact formulation.
As shown, e.g., in [17], finite-dimensional quantum theory as well as classical probability theory fit into this framework, as does box-world [7]. This allows all states on discrete sets of measurements that are non-signalling. The simplest non-trivial example of this is for elementary systems of type (2,2). The joint state space of two such systems includes PR-boxes. A key difference between box-world and quantum theory is that only the latter respects Tsirelson's bound.
3. Tsirelson's bound
The quantum correlation strength as quantified by the CHSH Bell inequality [13] is upper bounded by Tsirelson's bound [12, 25].
Definition 1 (Tsirelson's bound). Consider two systems A and B, with two choices of measurements (0 or 1) and two outputs each (a and b). Define the quantity

The theory governing the systems is said to satisfy Tsirelson's bound if for any states allowed by the theory.
A PR-box (also known as a non-local box) is designed to have S = 0 or 4, thus maximally violating the Tsirelson bound [23]. It is defined (up to relabellings of measurement choices and outcomes) to be a state where

and the local marginal states are uniformly random.
4. Information causality
Let there be two space-like separated parties, Alice and Bob which share an arbitrary no-signalling resource. Alice then receives a random bit string , which is not known to Bob. The bits ai are unbiased and independently distributed. At the same time Bob gets a random variable
, which is unknown to Alice. Alice is free to make use of her local resources in order to prepare a classical bit string
of length m which she sends to Bob. Bob, having received Alice's message, is then asked to guess the value of ab as best as he can. Let us denote Bob's guess by β. The efficiency of Alice's and Bob's strategy can be quantified by
where ISh(ai:β|b = i) is the Shannon mutual information between ai and β, computed under the condition that Bob has received b = i.
Definition 2 (Information causality). A theory is said to respect information causality if in the above game I ⩽ m for any allowed resource state.
It was shown in [22] that information causality implies Tsirelson's bound.
5. General entropy definition
We now recount certain results from recent research into how to quantify entropy in general probabilistic theories [4, 19, 24]. We shall, in particular, use a definition of entropy for general theories from [24] which is based on the Shannon entropy. This is highly analogous to how the von Neumann entropy generalizes the Shannon entropy to the quantum case. The intuition is that the von Neumann entropy is the minimal Shannon entropy over all measurements. Actually it is over all fine-grained measurements (explained below).
Note that one can in general define the Shannon entropy associated with a measurement e as .
Definition 3 (Entropy [24]). For every normalized state the entropy
is given by

denotes the classical probability distribution for the different outcomes of e and the minimization is over the set of all fine-grained measurements
.
above is defined to be the set of measurements which have no non-trivial fine-grainings. A fine-graining is a subdivision of one outcome into several different outcomes. A trivial fine-graining is one where the resulting outcomes do not have independent probabilities, or more formally, where the vectors representing the respective effects are proportional to the effect-vector associated with the original coarse-grained outcome.
The restriction to minimizing over is important. If one allowed coarse-grained measurements the entropy could always be reduced arbitrarily by grouping outcomes together into single outcomes. It is natural to draw the line at trivial fine-grainings since no more information is yielded by them.
The entropy can be interpreted as the minimal uncertainty that is associated with the outcome of a maximally informative measurement. It has some appealing properties: (i) H reduces to the Shannon entropy for classical probability theory and the von Neumann entropy in quantum theory, (ii) suppose that the minimal number of outcomes for a fine-grained measurement in
is d. Then for all states
,
and (iii) for any
,
and any mixed state
:
[24].
For a state of a bipartite system AB one defines the conditional entropy of A conditioned on B by [24]

with being the reduced state of
. If there are no ambiguities we drop the indices and we write H(A) instead of
and H(AB) instead of
, and so on.
Some properties that are satisfied in quantum theory (where this entropy reduces to the von Neumann entropy) are not necessarily satisfied for arbitrary theories. In box-world, for example the so-called strong subadditivity can be violated, as well as the subadditivity of the conditional entropy [24].
6. Data processing inequality
The DPI is a crucial property of entropy measures which is frequently used in proofs in classical as well as quantum information theory [14, 20]. DPI quantifies the notion that local operations cannot increase correlations. A standard formulation for the classical case is that H(X|Y ) ⩽ H(X|g(Y )), where X and Y are random variables which may be correlated, H(X|Y ): = H(XY ) − H(Y ), and g(Y ) is a function of Y only. The quantum DPI is the same, but with H denoting the von Neumann entropy.
We will use here the following generalized definition of DPI due to Short and Wehner [24].
Definition 4 (DPI). Consider two systems A and B. The data processing inequality is that for any allowed state and for any allowed local transformation

where H(·|·) denotes the conditional entropy of equation (3).
7. The main result
Our main result links the DPI with Tsirelson's bound.
Theorem 1. In any general probabilistic theory where the data processing inequality is respected, the Tsirelson bound is respected.
Proof. We here sketch the proof—see appendix for the details.
We use the fact that the entropy of definition 3 satisfies two properties: (i) H(A|B): = H(AB) − H(B) (we call this COND), and (ii) it reduces to the Shannon entropy for classical systems (we call this SHAN).
We prove that for any theory and entropy measure H jointly satisfying COND, SHAN and DPI, Tsirelson's bound holds (where DPI has been defined using H). This implies the main theorem.
The three conditions are not trivially applicable to restrict the resource state in van Dam's game, so we use them, within the framework of probabilistic theories, to derive certain more directly applicable lemmas, including: (i)
, where Ai denotes the ith party of a multi-party system A, (ii)
H(A) ⩾ H(A|B) with equality for product states, and (iii) for classical systems X, H(X|Y ) ⩾ 0. With these lemmas and some additional arguments, we show that information causality is respected, and thus, by [22], Tsirelson's bound.
8. Discussion
We have shown that the generalized DPI implies Tsirelson's bound. This addresses a question raised in [24], namely in what manner does enforcing generalized entropic relations restrict the set of possible theories. It also contributes to our understanding of why Bell violations in quantum theory respect Tsirelson's bound.
As indicated in the proof sketch, our quantitative results can be applied to more general entropy measures. In particular, for any entropy measure H and theory jointly satisfying COND, SHAN and DPI, we show that Tsirelson's bound holds. Thus one could alternatively have used, for example, the decomposition entropy of [24] in the statement of the main theorem as it satisfies SHAN and is defined to satisfy COND [24]. At the same time one may argue that while an operationally appealing definition of conditional entropy should automatically satisfy SHAN and DPI it is not clear why it should in general satisfy COND. COND may then be viewed as a restriction on states rather than a definition of conditional entropy.
One can compare our three sufficient conditions COND, SHAN and DPI to those used in [22] and [4], respectively. The entropic relations used in [22] to derive information causality were formulated in terms of a conditional mutual information I(A:B|C). (It is assumed that this can be defined in a more general setting, but no definition is given.) The conditions are that I(A:B|C) should: be symmetric under change of A and B, be non-negative (I ⩾ 0), reduce to the Shannon mutual information for classical systems, obey the DPI as formulated for mutual information and obey the chain rule I(A:B|C) = I(A:BC) − I(A:C). Arguably our three relations are more minimalistic and natural than those. Moreover, we show that the arguments apply to particular concrete definitions of entropy and that for at least two particular definitions of conditional entropy DPI alone suffices. Consider secondly [4]. There concrete entropy definitions are proposed and studied. The definitions are very similar to [24] although the framework is not a priori exactly identical. They define three properties in terms of conditional entropy as H(AB) − H(B), with H being the measurement entropy: (i) 'monoentropicity' (two particular different entropy measures always have the same value), (ii) a version of the Holevo bound and (iii) 'strong sub-additivity' (defined below). They show that those conditions imply information causality. They further note that conditions (ii) and (iii) can be derived from DPI defined in terms of the above conditional (measurement) entropy (more correctly they define it using mutual information I(A:B): = H(A) + H(B) − H(AB) but this is equivalent in this case). Assumption (i) is used to obtain what we here derive as equation (A.10). Thus it appears that one may alternatively summarize their result on information causality as follows: DPI (in terms of COND and measurement entropy) plus mono-entropicity implies information causality. This can be compared to our theorem 1; it is not so clear how to compare it to our more general theorem 2, as the latter does not refer to a specific entropy measure, but to any state space and conditional entropy measure jointly satisfying DPI, COND and SHAN.
DPI is related to a condition known as strong subadditivity (SSA) which states that H(A|CD) ⩽ H(A|C). SSA is implied by DPI since forgetting D is an allowed local operation. In the quantum case SSA also implies DPI, but this does not necessarily hold in other theories as the standard quantum proof relies on the specific quantum feature known as Stinespring dilation. In the extreme case of box-world, it was already known that SSA (and thus also DPI) is violated [24]. As an example, consider two classical bits x0, x1 and a gbit Z. The latter is a (2,2) system which can take any allowed distributions, i.e. its state space is the convex hull of four states wherein the two outcomes take defined values for each measurement. The classical bits are uniformly random but the gbit contains their values. Then H(x0|x1Z) = 1, whereas H(x0|Z) = 0, violating SSA [24].
It is an open question whether there are theories which satisfy DPI but have states not contained in quantum theory, since Tsirelson's bound is insufficient to rule out all non-quantum states. Understanding this and with what DPI needs to be supplemented in order to derive quantum theory fully are natural next steps.
Acknowledgments
We acknowledge comments by J Oppenheim, A Short and S Wehner on an earlier draft, advice on references by V Scarani, as well as funding from the Swiss National Science Foundation (grant no. 200020-135048) and the European Research Council (grant no. 258932). This work was carried out in connection with DL's master's thesis at ETH Zurich.
Similar results have been obtained independently in [1] by Al-Safi and Short.
Appendix.: Proof of the main theorem
The main theorem is a direct corollary of a more general theorem, theorem 2, which we state and prove in this section. Crucially, theorem 2 does not refer to a specific entropy measure such as the measurement entropy defined above.
We require three definitions to state this theorem.
First we redefine DPI, now defined without reference to a specific entropy definition.
Definition 5 (DPI). Consider two systems A and B. The DPI is that for any allowed state and for any allowed local transformation

Definition 6 (Conditional entropy (COND)). The conditional entropy H(A|B), however it is defined, must for all allowed states on AB satisfy

Definition 7 (Reduction to Shannon entropy (SHAN)). The entropy H must reduce to the Shannon entropy for classical systems.
Our statements are restricted to the generalised probabilistic framework, as described in the introduction of this paper. We shall be making use of two non-trivial but operationally well-motivated types of transformations associated with that framework: adding and removing systems. An (independent) system in state is added by the map taking any
to
. A system is removed by taking the marginal distribution on the other system(s), as described in the introduction. We shall make use of the fact that this map acts to take the removed system B to the vacuum system V . The only normalized state of the vacuum is
(this can be seen from the equivalent definition of the marginal state used, e.g., in [6]). Thus, and this is another equation we shall find useful,
.
We shall also be assuming that the entropy measure is operational, i.e. is uniquely determined by the statistics of the experiment under consideration. Thus it is for a given setup determined by the state of the systems under consideration. More subtly, H moreover cannot depend on the order in which the state-spaces of the subsystems are composed, as this order is arbitrary; different observers describing the same experiment can make different choices here. Thus H(AB) must be invariant under the interchange of systems A and B.
We are now ready to state the theorem:
Theorem 2. For any probabilistic theory and entropy measure H satisfying COND, SHAN and DPI, Tsirelson's bound holds.
Before proving theorem 2 we note that the main theorem (1) is directly implied by this statement as the entropy H referred to there satisfies COND and SHAN.
Before proving theorem 2, we prove some lemmas which we shall need and which may be of interest in themselves.
Lemma 3. COND and DPI imply the relation

for any , where Ai denotes the ith party of the total system A1,...,An.
Proof. Consider first n = 2. By COND we have

By DPI this is greater than or equal to 0.
To generalize the argument to n > 2, let A2 be replaced by A2,...,An in the previous equation. Then by the same argument

Now we can apply the previous argument to the term H(A2,...,An|γ) to get

This process is then repeated iteratively to recover . □
Lemma 4. For product states , COND, SHAN and DPI imply the relation

Proof. We first use COND and SHAN to show that H(A|V ) = H(A) for any system A. This follows from the following:



(Here COND implies the first line. As V is classical and with only one measurement outcome, SHAN implies H(V ) = 0; as mentioned in the beginning of the appendix.)
We now prove the equality of the lemma by separately proving the two corresponding inequalities in both directions. Note first that

for any state. To see this, consider the transformation T that takes B to the vacuum system (i.e. the transformation that removes B as described in the introduction to the appendix). Then, using DPI,

Consider, secondly, the inequality in the other direction, restricting ourselves to the case of product states only:

This is true because , where the last step uses DPI for the transformation that creates
from the vacuum state (i.e. the transformation that adds
B as described in the introduction to the appendix).
Combining equations (A.8) and (A.9) proves the claim. □
Lemma 5. DPI, SHAN and COND imply that for all classical systems X,

Proof. To prove the lemma via DPI we shall use the fact that the extremal states of classical systems can be cloned [6]. More specifically, we shall make use of the fact that for a classical system XA in state , where the
are pure, and another classical system XB of the same dimensionality in any given independent pure state
, there exists a map TC such that
.
We shall consider a three-party system Y
XAXB, where Y is the only non-classical sub-system. The idea is that given an arbitrary state on Y
X: = Y
XA, we can always bring in another independent subsystem XB and perform a cloning operation so that XB becomes a copy of XA. We may then apply DPI to the cloning transformation TC applied to XA and XB. We call the states before and after the cloning as and
, respectively.
By DPI we then have

Note now that the left-hand side can be simplified. COND together with equation (A.4) imply that H(AB) = H(A) + H(B) for independently prepared A and B. This can be applied here because XB is initially in an independent state, yielding

Accordingly

We also note that the marginal state on Y
XA is unchanged by the cloning, i.e. , so we may for simplicity write that for the state after the cloning,

In the following, unless stated otherwise, we consider the state after the cloning only.
Applying equation (A.2), i.e. COND, to equation (A.12) and undertaking some rearrangements yields

Moreover, SHAN implies that H(XB|XA) = 0. Thus

Note that since XA and XB are operationally indistinguishable after the cloning, H(XA|Y XB) = H(XB|Y XA). Thus we have

By DPI

Thus, still after the cloning, we have that

But since the state of XAY is unchanged by the cloning transformation, this implies that the equation holds also for the (arbitrary) initial state of XAY . Recall that we used XA to label the classical system X. We have thus shown that H(X|Y ) ⩾ 0 for an arbitrary initial state on XY. □
Lemma 6. COND, SHAN and DPI imply the relation

where the quantities are as defined in the information causality game ( is the classical n-bit string given to Alice, B is the non-classical resource and
is the classical m-bit message sent to Bob).
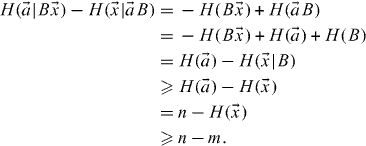
The first line follows from COND. The second line is due to the combination of equation (A.4) and equation (A.2) and recalling that and B are independent. The third line uses equation (A.2) again. The fourth line follows from equation (A.8). The fifth and sixth lines follow from the definition of the game as well as elementary properties of the Shannon entropy, which can be exploited due to SHAN.
It follows by applying equation (A.10) to the left-hand side that . □
We now put together the pieces to prove theorem 2:
Proof of theorem 2. By lemma 6, we have

By lemma 3 this implies that
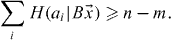
By DPI we accordingly have that for Bob's guess
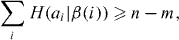
where, by SHAN and the fact that ai and β(i) are both classical, H refers to the Shannon entropy.
This implies information causality, as ISh(ai:β(i)) = H(ai) − H(ai|β(i)), so
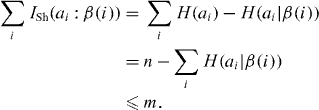
Recall that information causality implies Tsirelson's bound. □