Abstract
It is well-known that crowded environments affect the stability of proteins, with strong biological and biotechnological implications; however, beyond this, crowding is also expected to affect the dynamic properties of proteins, an idea that is hard to probe experimentally. Here we report on a simulation study aimed at evaluating the effects of crowding on internal protein dynamics, based on fully all-atom descriptions of the protein, the solvent and the crowder. Our model system consists of ubiquitin, a protein whose dynamic features are closely related to its ability to bind to multiple partners, in a 325 g L−1 solution of glucose in water, a condition widely employed in in vitro studies of crowding effects. We observe a slight reduction in loop flexibility accompanied by a dramatic restriction of the conformational space explored in the timescale of the simulations (∼0.5 µs), indicating that crowding slows down collective motions and the rate of exploration of the conformational space. This effect is attributed to the extensive and long-lasting interactions observed between protein residues and glucose molecules throughout the entire protein surface. Potential implications of the observed effects are discussed.
Export citation and abstract BibTeX RIS
Introduction
Cellular environments are crowded by solutes and macromolecules of all sizes, creating conditions far from those present in typical in vitro experiments [1]. The solute concentration in the cytoplasm of E. coli cells is estimated to be in the order of 300–400 g L−1 [1, 2]. This can be conceptualized visually and numerically in recent computational works [2, 3] to reach the conclusion that intracellular solutes must inevitably experience non-specific, unintended interactions with each other. Especially for macromolecules, such interactions are expected to introduce significant alterations in the thermodynamics and kinetics of the processes they are involved in. In the case of proteins, some of these alterations have been observed and quantified through experiments in which a property is quantified in the presence of increasing quantities of a crowding agent such as small sugar moieties, dextrane, PEG, Ficoll or other proteins. One of the most important and generalized findings is that the crowding agent usually improves the stability of globular proteins to the extent that it can even induce folded states on chemically denatured proteins and on natively unfolded proteins [3–10]. These stabilizing effects are typically in the order of a few kcal/mol, but they represent an important contribution and are expected to be relevant in vivo because proteins are only marginally stable [4]. On the other hand, structural stabilization and crowding are expected to alter the landscape of the conformational space accessible for motions, potentially compromising dynamic features important for protein function and regulation and for protein–protein interactions.
Although little is known about the underlying processes that drive crowding effects, most experiments suggest that they are rather non-specific, supporting the notion that repulsions and steric (i.e. entropic) effects are dominant. However, recent works reported approximately similar contributions from repulsions and chemical (i.e. enthalpic) interactions between proteins and crowders, although no specific interactions were observed with the crowders [5, 6]. Unfortunately, atomistic investigations of the impact of crowding on protein structure and dynamics are inherently difficult: in x-ray studies crystal packing does not leave space for crowding assays, whereas in NMR studies the increase in viscosity produced by high crowder concentrations broadens signals, eventually rendering them undetectable. As an example, NMR studies could not go beyond 100 g L−1 solutes, which would be desirable to achieve the crowding levels akin to those inside cellular environments [5–7].
As in many experimentally intractable phenomena, molecular dynamics simulations are a powerful alternative to study the problem of interest at the atomistic level. Indeed simulations have been carried out on a few proteins under cell-like crowded conditions, helping us to understand some of the effects of crowding [3, 8–13]. Among these works, most treated the crowder molecules as coarse, big spheres that cannot account for the effects of chemical interactions. Only the most recent simulations have employed fully atomistic descriptions of the systems [3, 9, 10], starting to reveal the importance of enthalpic effects together with recent experiments [5, 6]. However, none of these works studied the effect of crowding on the internal protein dynamics. In order to start filling this gap, we studied the effect of glucose crowding at 325 g L−1 on the dynamic features of ubiquitin through all-atom MD simulations.
Ubiquitin stands as an interesting workhorse protein for studying the effect of crowding on protein structure and dynamics because both aspects have been studied thoroughly through experiments and simulation [14–26]. Such studies have disclosed how structural fluctuations are intimately linked to the protein's capacity to interact with other proteins to achieve its primary function of targeting their fates in the cell, in-between what conformational selection and induced fit models predict [20, 22, 27–29]. Also, ubiquitin has been the subject of a few experimental studies under crowded conditions [5, 30, 31]; more specifically, it has been reported that wild type ubiquitin and three destabilized mutants of this protein gain stability in solutions crowded artificially with glucose or dextrose and that the amount of gained stability is similar for the wild type and destabilized mutants, and for glucose and dextrane, suggesting that the operating mechanism is unspecific [30]. Also, a very recent work revealed that crowding effects on ubiquitin are mediated by unspecific chemical interactions and repulsion effects in roughly similar amounts [5].
Our extensive knowledge on ubiquitin dynamics and its role in target recognition is a picture derived from experiments in dilute conditions and simulations in explicit water. However, no study has assessed the effect of crowding on the protein's dynamic features at the atomistic level. With this motivation in mind, we proceeded to run and compare simulations of ubiquitin in pure water and in 325 g L−1 glucose as a starting point to study this matter. Glucose at 325 g L−1 was chosen to mimic a crowded medium because (i) despite still being far from true biological conditions, it is a popular solute crowder used in experimental studies, and (ii) because such studies have shown that it exerts several effects on proteins including stabilization of the protein fold. Moreover, (iii) accurate atomistic force fields for molecular dynamics simulations of glucose are available [32] allowing one to obtain a realistic model of a simple crowded environment for ubiquitin.
Methods
Molecular dynamics simulations
Simulations were run with the NAMD [33] code using the amber99SB [34] force field for the protein, TIP3P [35] parameters for water and Glycam06 [32] parameters for α-D-glucose. The structure of human ubiquitin in PDB ID 1D3Z [36] (model 1) was used as a starting point for minimization, equilibration and production in both simulations. For the simulation of ubiquitin in water, the molecule was solvated in a TIP3P box (315 396 Å3); whereas for the simulation under crowded conditions, the molecule was first added to 317 glucose molecules using the Packmol [37] program, and then solvated with TIP3P water molecules. After minimization and equilibration the volume of the box was 291 135 Å3, resulting in an average concentration of 325 g L−1.
Principal components analysis and projection of the trajectories
To build the reference frame for trajectory projection, principal components analysis (PCA) was carried out on the covariance matrix of Cα positions for residues 2–70 of 72 high-resolution x-ray structures of human ubiquitin aligned to model 1 of PDB ID 1D3Z [36]. This is the same strategy used by others [22, 27], but is based on a larger set of structures. This set includes free monomers, covalent oligomers and complexes with binding partners, all solved at a resolution equal or better than 2.5 Å (the list of PDB codes and PDB titles can be found in table S1 (available from stacks.iop.org/PhysBio/10/045006/mmedia)). The projection of the trajectories on the reference frame was also preceded by an alignment to model 1 of PDB ID 1D3Z. The sum of squared PCA loadings (i.e. eigenvalues) 1 and 2 shows that the structural variability that spreads the x-ray structures on the reference frame arises from sequence segments 6–11, 33–36 and 46–49 (figure S2B (available from stacks.iop.org/PhysBio/10/045006/mmedia)), which indeed show different conformations in the set of structures (root mean squared fluctuations (RMSF) from structures in figure 1(A)).
Figure 1. Functional flexibility of ubiquitin as derived from x-ray structures and MD simulations. (A) RMSF observed in the 72 x-ray structures on which PCA was carried out. (B) Average number of interactions observed for each ubiquitin residue with all other proteins in the 72 x-ray structures used for PCA. (C) RMSF derived from simulations of ubiquitin in water (black) and 325 g L−1 glucose (red). Panels (D), (E) and (F) color-code the same information on the structure of ubiquitin (increasing from blue to red): average number of contacts (D) and flexible regions as determined from the RMSF in water (E) and in 325 g L−1 of glucose (F). The C-terminus (residues 71–76) is not reported because it is missing some structures due to its high flexibility (trimmed in panels (D)–(F)).
Download figure:
Standard image High-resolution imageCalculation of free-energy landscapes
Free-energy landscapes were built from the trajectories projected on the reference frame by binning the projection into a grid and counting the number of frames inside each cell of the grid (Ni) to compute its free energy according to:

where N0 corresponds to the most populated bin, which thus sets the free energy offset.
Interactions between ubiquitin and other proteins in x-ray structures
The average number of ubiquitin–partner interactions per residue was approximated as the average (through all x-ray structures that contained a protein interacting with ubiquitin) number of Cα atoms of ubiquitin-bound proteins that are within 5 Å of each Cα atom of ubiquitin.
Calculation of NMR parameters from MD trajectories
N–H order parameters (s2) and residual dipolar couplings (RDC) were computed from the trajectories using equations reported in the literature. The trajectory is aligned to model 1 of PDB ID 1D3Z and the normalized N–H vectors u are retrieved for each peptide bond in each frame of the aligned trajectory. The calculation of s2 is done as described elsewhere [38] assuming full decay of the autocorrelation function for u during the time of the trajectory:

where the average 〈ui,α ui,β〉 is computed over the whole trajectory.
The N–H RDC were computed for each alignment medium for which data are available, as described by Showalter et al [39]. Briefly, for each medium an alignment vector A = (Axx, Ayy, Axy, Axz, Ayz) is fit that minimizes the difference between the experimental RDC data (vector Dexp with as many elements as N–H peptide bonds for which data are available in that alignment medium) and the back-calculated RDC values (Dback of same size) in a least-squares sense. The back-calculated data is Dexp = M × A, where M is a matrix derived from the trajectory formed by one row of 〈ux2〉 − 〈uy2〉, 〈uy2〉 − 〈uz2〉, 2〈uxuy〉, 2〈uyuz〉 and 2〈uyuz〉 values for every N–H pair for which data are available. The least-squares problem (M × A – Dexp → min) is solved by singular value decomposition as described in detail in that same work [39].
Analysis of contacts between water or glucose molecules and the protein
Interactions between water or glucose molecules and the protein were investigated by searching for contacts between the small molecules and the protein. Two atoms were considered to be in contact when the distance between them was shorter than 1.1 times the sum of their Van der Waals radii, as optimized in recent works [40, 41].
Root mean squared fluctuations of Cα atoms in protein ensembles
RMSF were computed for each Cα atom as:
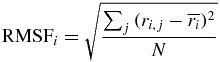
where ri denotes the position of the Cα atom i, and j runs through the N frames of simulated time or x-ray structures. Cα atoms 2 to 70 were considered for the ensemble of x-ray structures and Cα atoms 1 to 76 for the simulations. Calculations were done after alignment to a reference structure (i.e., model 1 of 1D3Z).
Kinetic description of basins in the conformational landscapes
The analysis proposed by Hess [42] was carried out on the basins observed in the conformational landscape of each simulation to describe them in terms of their deepness and roughness. First, ∼20 ns long sections of the trajectories were identified in which the protein remained inside each basin according to their projections on the two-dimensional reference frame built from the x-ray structures. For each sub-trajectory, PCA was carried out to obtain the most important fluctuations inside each basin; the first and second eigenvalues (λ) were used to compute the force constants k that defines the harmonic well in the direction of the two most important eigenvectors, using the formula:

where kB is Boltzmann's constant and T is 300 K. The autocorrelation functions were computed for the first principal components and their first decaying parts were fitted to obtain the reported τ parameters. The internal friction-like coefficient η is then:

which measures the roughness of the basin completing its description together with the harmonic well constant k.
Results and discussion
Simulation of ubiquitin dynamics in water
The dynamic features of ubiquitin in dilute solution have been vastly studied by NMR, revealing nano- to micro-second timescale motions that are important for target recognition [20, 22, 27–29]. These experimental observations were complemented with molecular dynamics simulations in several works to further dissect the contributions of conformational selection and induced fit mechanisms to target binding [27, 28, 43]. Of particular importance, 0.5 to 1 µs long simulations performed with amber99SB, a highly accurate force field that fairly reproduces NMR dynamic data, showed that free ubiquitin is constantly exploring conformational states that resemble those observed in x-ray structures of the protein complexed with different partners [27, 28]. Based on these works, we first performed a reference 500 ns long simulation of free ubiquitin in water using the amber99SB force field. As reported for previous simulations, ours also reproduces fairly well the experimental order parameters available from relaxation and RDC data [14] (figure S1A (available from stacks.iop.org/PhysBio/10/045006/mmedia)) and the RDC measured in 39 alignment media [17, 18, 25, 44–46] (figure S1B (available from stacks.iop.org/PhysBio/10/045006/mmedia)). The RMSF profile derived from the simulation (black curve in figures 1(C) and pictorial representation (E)) follows that observed in a set of x-ray structures of ubiquitin bound to different partners (figure 1(A)), both pointing at increased dynamics of the loops involved in the recognition of ubiquitin's binding partners (figures 1(B) and (D)).
All in all, this analysis shows that the amount of flexibility observed in the trajectory is consistent with experimental data in dilute solution and that flexible regions of the protein are important for its ability to bind different partners. However, the analysis does not reveal any information about the conformational space accessible to the protein through collective motions as a result of such flexibility. In order to explore this we projected our simulation on a two-dimensional reference frame built by mapping through principal components analysis the structural variability observed in 72 experimental x-ray structures of human ubiquitin under different scenarios (figure S2A (available from stacks.iop.org/PhysBio/10/045006/mmedia), where each red point is an x-ray structure). This method has recently been introduced to analyze simulation trajectories and ensembles for which no specific reaction coordinate can be defined a priori [22, 27, 28]. Briefly, this method combines the collective fluctuations of the most variable regions of the protein into two new variables, i.e. the principal components 1 and 2, which account for as much fluctuation as possible. Projection of the Cα coordinates of our trajectory on this two-dimensional frame (figure 2(A) in blue, where only one frame was plotted every 1 ns for simplicity) shows that the protein explores the whole range of conformations sampled by the x-ray structures, covering smoothly the conformational space. Figure 2(B) is a simpler and more informative representation of the projection, in which the density of frames projected on a grid was converted into a free energy landscape relative to the deepest well. The largest barriers separating these states are around 1 kcal/mol, indicating a nearly flat energy surface consistent with the very fast exchange observed between conformational states and with the fast dynamics determined by NMR.
Figure 2. The conformational landscape sampled by ubiquitin in water and 325 g L−1 glucose. (A) and (C) 500 frames of the 500 ns trajectories in water (blue in (A)) and glucose (green in (C)) projected on the reference frame built through PCA of x-ray structures (red points). (B) and (D) Free energy landscapes computed from both MD trajectories relative to the lowest energy point in each of them, color-coded as shown on the bars in kcal/mol.
Download figure:
Standard image High-resolution imageUbiquitin dynamics in 325 g L−1 glucose
In the next step we investigated a 500 ns long MD trajectory of ubiquitin in a solution crowded at 325 g L−1 of glucose. For this simulation we combined the amber99SB force field [34] to describe the protein and the Glycam06 [32] force field to describe glucose molecules. Our setup leaves a random distribution of water and glucose molecules in a ratio of about 20:1 around the protein molecule; details on the system setup and simulation are given under methods.
A comparison of the RMSF in 325 g L−1 glucose against the values computed in water (red versus black in figure 1(C), respectively) shows that the amplitudes of loop motions are slightly reduced under crowded conditions during the timescale of the simulations. Notably, the effect on RMSF is not as drastic as that observed in a previous simulation of myoglobin under much more crowded, near-dry conditions at almost 90% w/w sucrose [47, 48]. As a result of the mild reduction in loop flexibility, the RMSF and s2 values of the loops homogenize in the crowded condition, indicating similar mobility at 325 g L−1 crowder concentration. Importantly, and as shown in figure S4 (available from stacks.iop.org/PhysBio/10/045006/mmedia), this equalizing effect is also seen on the N–H order parameters, which can be obtained experimentally and compared to test our findings. One final point worth noticing is that the root mean squared velocities of Cα atoms are roughly similar in both simulations (data not shown) indicating that the effect of crowding is on the amplitudes of local atomic fluctuations but not on the atoms' speeds.
In order to explore the impact of crowding on collective motions and the conformational landscape explored by the protein, we projected the simulation on the two-dimensional reference frame built above. Projection of one frame every 1 ns (figure 2(C)) shows that the protein has not explored the same conformational space as in water during the same time length. Instead, it is restricted to small excursions slightly away from its initial conformation, always staying within the same basin. This is also evident in the time evolution of the conformational space sampled in figure 3. The finding of a strong restriction in the size of the explored conformational space with only a minor reduction in RMSF indicates that local fluctuations take place to a similar extent under both conditions but that collective motions (i.e. transitions between basins) have been compromised or more likely slowed down in the crowded condition.
Figure 3. Crowding slows down the kinetics of exploration of the conformational space. Columns show the conformational space sampled at increasing time points of the simulations in water (left) and in 325 g L−1 glucose (right). The time corresponding to each free energy plot is indicated on the bottom left of each panel.
Download figure:
Standard image High-resolution imageExploration of basins in both simulations
In only 40 ns of simulation time the protein simulated in water has escaped from the initial energy well and has already explored most of the conformational space that it will sample during the rest of the simulation (figure 3, left). In contrast, the simulation in 325 g L−1 glucose is still trapped around its starting point at 300 ns (figure 3, right) and has made only one unsuccessful attempt to visit an alternative conformation at around 400 ns (figures 2 and S3 (available from stacks.iop.org/PhysBio/10/045006/mmedia)). We also observe that the speed at which the protein explores the conformational space is slower in the crowded condition than in water. In order to quantify this, we studied the rate at which the conformational basins are explored based on a formalism proposed by Hess [42] to model the 'diffusion' of a protein inside a conformational basin in terms of the internal friction coefficients (η) and harmonic force constants (k) characteristic of the basin. We analyzed 20 ns long sections of the trajectories in which the protein remained inside each of the two basins of the simulation in water and in the single basin observed in glucose solution. The parameters obtained for the two first principal components of motion inside each basin are given in table 1. The two basins observed in water are characterized by similar harmonic force constants, whereas the basin observed in the crowded condition features a ∼5 times stronger k meaning a deeper and sharper energy well. The later is also rougher with η being an order of magnitude larger, meaning an increased 'friction' and a much slower exploration of the basin in the presence of crowder.
Table 1. Analysis of internal dynamics within each conformational basin.
λ (Å2) | k (kJ mol−1 nm−2) | τ (ps) | η (amu ps−1) | |
---|---|---|---|---|
Water (left basin) | ||||
PC 1 | 8.84 | 28.2 | 9420 | 2.66 105 |
PC 2 | 3.78 | 65.9 | 5860 | 3.86 105 |
Water (right basin) | ||||
PC 1 | 6.49 | 38.5 | 1350 | 5.19 104 |
PC 2 | 3.46 | 72.1 | 681 | 4.91 104 |
325 g L−1 glucose | ||||
PC 1 | 1.46 | 170 | 7950 | 1.36 106 |
PC 2 | 0.69 | 360 | 3700 | 1.33 106 |
In brief, our results predict that crowding slows down the internal dynamics by exerting an increased 'internal friction' and possibly by imposing higher energy barriers around the conformational basins thereby reducing the rate of exchange between them. Importantly, the effect is important for large scale motions but not that much for atomic fluctuations. Similar ideas implying that crowding slows down internal motions have been suggested by experimental observations in the last few years [49, 50]. Also, recent computational and experimental studies showed that the confinement of a protein inside a micelle reduces its internal dynamics due to interactions with the surfactant [51, 52]. With all this evidence, the notion that internal protein dynamics are affected by crowding starts to gain support.
Extensive interactions between glucose molecules and ubiquitin
Considering the high concentration of glucose molecules in the simulation box, encounters between these molecules and the protein are very likely to occur. Indeed, visual inspection reveals extensive associations of several glucose molecules with the protein, resembling the multimolecular complexes observed in a recent all-atom simulation of the cytoplasm of E. coli [3]. In the absence of crowders, the protein interacts with an average of 289.6 water molecules at any given moment; whereas in 325 g L−1 glucose, this decreases to an average of 120 water molecules due to an average of 73.1 glucose molecules that attach to the protein surface. This means that every ∼169.6 water molecules that are excluded from the surface, ∼73.1 glucose molecules are attached, resulting in a ratio of ∼2.3 water/glucose present at the protein surface. This ratio is consistent with the larger area of glucose and shows how interactions between the crowder and the protein result in desolvation of its surface, as observed through simulations and experiments for near-dry conditions [47, 48, 53] but to a lesser extent. Notably, five glucose molecules never leave the protein surface along the whole trajectory. This does not imply constant interaction of the glucose molecules with the same protein residues; rather, the glucose molecules shuffle interactions to different atoms of the protein wandering around its surface. For example, there is a glucose molecule that interacts with the first loop of the protein, switching hydrogen bonds between its hanging alcohol groups and different atoms of the protein during the whole simulation (figures 4(D) and (E)).
Figure 4. Interactions between ubiquitin and water/glucose molecules. (A) Average number of water molecules in contact with each residue at any given moment along the simulations with (blue) and without (red) crowders. (B) Average number of glucose molecules in contact with each residue in the simulation in 325 g L−1 glucose. (C) Relative solvent accessibility for each residue, as calculated with ASAView [58]. (D) and (E) Typical sugar–ubiquitin interactions during MD. The time evolution of relevant distances between three different oxygen atoms of one glucose molecule and different ubiquitin atoms of the first loop is reported in panel (D), while in (E) their spatial distribution is reported using the same color code.
Download figure:
Standard image High-resolution imageAnalyses per residue (figures 4(A)–(C)) reveal homogeneous desolvation and glucose binding along the exposed parts of the protein, suggesting extensive enthalpic perturbations that average smoothly on the protein surface. This picture is in line with the recent finding that unspecific interactions are an important component driving crowding effects on top of the already known steric effects. Regarding desolvation, perturbations of the hydration structure and dynamics were predicted in a recent simulation meant to assess the effect of protein rather than small-molecule crowders [10]. In the case of glucose molecules, however, the perturbation of hydration structure and dynamics on the first water shell seems stronger, which can be attributed to the much smaller size of glucose and its higher density of polar groups. In our interpretation, glucose molecules bound to the protein and water molecules trapped in-between drag the exposed residues. Since the interactions are not specific, the effect is generalized and affects all collective motions making them less likely to happen; in other words, slowing them down. Further work is underway to better understand these effects of crowding on the internal protein dynamics, and to evaluate the role of protein–glucose, protein–water and glucose–water interactions.
Possible physiological roles of crowding
Although the constituents of the cellular medium are chemically varied and not simply glucose rings, they contain exposed polar and charged groups capable of establishing electrostatic interactions, salt bridges and hydrogen bonds with protein surfaces, which are largely polar and charged. In our view, based on these and others' results, all these transient, unspecific interactions average out to a constant enthalpic and entropic perturbation of the protein surface. This perturbation would imply both a thermodynamic stabilization of the protein fold and a slow down in all large-scale kinetic processes thus slowing down unfolding rates too. In this regard, our observations are in agreement with the experimental report that crowding enhances the native structure of proteins [54, 55] and that part of this stabilizing mechanism acts on the folding pathways themselves [12]. On top of this, our finding that collective motions are slowed down allows us to propose that crowding agents can stabilize proteins by simply trapping the native conformations, i.e. through a kinetic contribution. Similar ideas were suggested by different experimental and computational evidence [56, 57]; thus this possibility deserves further exploration.
Notably, these effects would also stabilize protein complexes, as well as complexes composed of different kinds of macromolecules as reported in a recent all-atom simulation of E. coli's cytosol. This might be of little importance for strongly interacting proteins but would be important for proteins involved in interactions defined as 'transient' by experiments. In particular this can explain why some interactions predicted by genetic evidence are not confirmed by in vitro experiments. Stabilization of protein–protein interactions by crowders would have profound implications for many proteins, including ubiquitin itself. Moreover, crowding could have an effect on the relative weight of induced-fit and conformational selection mechanisms that guide protein–protein recognition.
Finally, we can speculate that the marginal stability observed for proteins in solution arises from the fact that they have evolved to work in crowded, stabilizing environments. Under this scenario, potential proteins that are too stable in dilute solution would be unnecessarily stable in the cellular medium and possibly too rigid to function properly.
Conclusion
Crowding effects are very important for life as we know it, as they influence the thermodynamics and kinetics of cellular chemistry, and in biotechnology they can be exploited to stabilize protein samples and in protein refolding protocols. We have herein investigated the effect of glucose crowding on the internal dynamics of a protein, providing a starting point to better describe the interplay between the protein–glucose, protein–water and glucose–water interactions on the protein surface at the atomic level, and how they influence protein dynamics and stability.
Finally, our results back up the notion that crowding might be an element with which cells manipulate protein dynamics affecting their folding, function and regulation. As increasingly recognized by our findings and by several recent works, more realistic insight into the biological physics and chemistry of the cell might be obtained by including crowders in experimental and computational research.
Acknowledgments
We thank Francesco Piazza (CNRS Orleans, France) for useful discussions and comments on the manuscript. LAA thanks EMBO and the Marie Curie Actions for a postdoctoral EMBO Long-Term fellowship.