Abstract
India is one of the world's largest food producers, making the sustainability of its agricultural system of global significance. Groundwater irrigation underpins India's agriculture, currently boosting crop production by enough to feed 170 million people. Groundwater overexploitation has led to drastic declines in groundwater levels, threatening to push this vital resource out of reach for millions of small-scale farmers who are the backbone of India's food security. Historically, losing access to groundwater has decreased agricultural production and increased poverty. We take a multidisciplinary approach to assess climate change challenges facing India's agricultural system, and to assess the effectiveness of large-scale water infrastructure projects designed to meet these challenges. We find that even in areas that experience climate change induced precipitation increases, expansion of irrigated agriculture will require increasing amounts of unsustainable groundwater. The large proposed national river linking project has limited capacity to alleviate groundwater stress. Thus, without intervention, poverty and food insecurity in rural India is likely to worsen.
Export citation and abstract BibTeX RIS

Original content from this work may be used under the terms of the Creative Commons Attribution 3.0 licence. Any further distribution of this work must maintain attribution to the author(s) and the title of the work, journal citation and DOI.
1. Introduction
Agriculture is a significant part of India's social and political economy. While most of India's agricultural production processes are small scale in nature, in total they account for 20% of India's GDP and are India's largest employers. Moreover, the agriculture sector is the primary food supplier for India's 1.2 billion people. India is also one of the world's largest agricultural producers, and exports close to $39 billion in raw agricultural products and over 4.4 million tons of milled rice annually [1, 2]. Much of Indian agriculture is heavily dependent on irrigation. Beginning in the 1960s, with the onset of the Green Revolution, India saw a significant increase in groundwater irrigation [3]. This increase was primarily driven by the emergence of atomistic or personal irrigation systems and the use of subsidized power to pump groundwater from individual tube wells [3]. Through this process, approximately 90 million rural households have come to directly depend on groundwater irrigation [4]. Between 1970 and 2004, while crop area remained fairly stable, irrigated area saw a rapid increase with groundwater extractions accounting for 70%–80% of the value of agricultural production (5, figure S1). This underscores the important role that groundwater irrigation has played in supporting upward trends in yields and productivity.
Increased use of groundwater irrigation has led to widespread over-abstraction of groundwater resources [6], which is unsustainable in the long term. Since 1980, groundwater levels have dropped from 8 meters below ground level (mbgl) to 16 mbgl in northwestern India and from 1 to 8 mbgl in the rest of the country [6–8]. Northwestern India lost 109 km3 of groundwater between 2002 and 2008 [6], which is an order of magnitude larger than the groundwater depletion experienced by California's Central Valley during the same period [9] and twice the volume of India's largest surface water reservoir [6]. Previous research has demonstrated that groundwater declines can lead to increases in poverty and threaten food production—especially for rural households [10, 11]. This directly affects small-scale farmers, who typically own <2 ha of farmland, control the majority of the landholdings in India and produce 41% of India's food grains [12]. These farmers use groundwater to irrigate half their land and are likely to be the hardest hit by continued declines in groundwater, thus adding to their already high vulnerability. Consequently, the sustainable use of groundwater into the future remains a serious concern for India, especially given the substantial number of rural households dependent on it for their sustenance. Previous studies in the fields of hydrology [13, 14] and economics [15] have individually assessed the future of groundwater-based agriculture in India; however, each of these fields misses key elements. Previous hydrology work has not accounted for the dynamic behavioral response of farmers to changing climate, while econometric studies have failed to account for biophysical constraints on water supplies. Researchers and policymakers need a modeling system that can account for both human irrigation decisions and the physical water supply in order to understand how climate changes may affect unsustainable groundwater (UGW) use—defined as any groundwater abstraction in excess of recharge—groundwater levels and agricultural production.
Figure 1 outlines the major components of both the human and physical water systems, and the dynamics within and between the two systems that have the potential to impact groundwater use and crop yields in India. In this study, we restrict our focus on how climate change will drive human irrigation decisions, and in turn how both those human decisions and the physical changes in climate will alter both crop water requirements and available water resources. This approach requires integrating an econometric model with a process-based hydrology model (figure 1), and allows us to quantify not only how climate change will alter each system individually, but how it will affect the entire coupled system. Such a multidisciplinary approach is required for study of the widely varying spatial differences in water resources and crop production across a country that is both experiencing a significant water crisis and is home to a third of the world's extreme poor, who primarily rely on agriculture for their livelihoods [16]. The combination of both models is critical for India in order to better plan for the future, as well as assess the role that adaptation responses and policy measures play going forward. One such policy initiative proposed by the Government of India is to move 178 billion m3 yr−1 of water across river basin boundaries [17]. This national river linking project (NRLP) has been proposed as a solution to groundwater stress by increasing irrigated agriculture through surface irrigation and artificial groundwater recharge. Better understanding of future irrigation water demand and availability, with emphasis on UGW dependence, is critical to assess such policies and formulate effective strategies to adapt to climate change.
Figure 1. Conceptual framework for coupled human-physical water system modeling of India's groundwater future. Climate change, population growth, technological advances, and markets (including agricultural product prices, trade, and GDP) directly impact multiple components of both the human and physical water systems. Components within these systems respond individually to external changes, but also impact each other. Hydro-infrastructure is a key component of both the systems. Arrows between the systems show important linkages that should be considered when studying the future of groundwater in India. Black arrows and components in shaded boxes are modeled endogenously in this paper's analysis; gray arrows and outlined components are accounted for either through model inputs or through assumed continued historical trends. This conceptual framework can be used to develop model systems that endogenize additional or alternative components than those assessed within this paper.
Download figure:
Standard image High-resolution image2. Methods
We make use of detailed crop-wise agriculture and weather data spanning 36 years from 1970 to 2005 for all the districts in the main agricultural states of India, and a panel data regression to estimate the relationship between inter-annual variation in monsoon climate variables and district-level irrigation decisions. This relationship is used to generate projections of irrigated area in response to climate change. The hydrology model then simulates irrigation water demand and supply from surface and renewable- and non-renewable groundwater based on these projections and future climate inputs. A detailed account of the methods and data is available in the supporting information. Our coupled-model approach allows us to assess India's future dependence on UGW and its impacts on groundwater-based agricultural production. We also quantify the effect of the proposed NRLP in mitigating groundwater stress.
2.1. Econometric model: human system
We project irrigated area for six major crops in India—the staple cereal crops rice and wheat (the focus of the Green Revolution); coarse cereals maize, sorghum and barley; and a high-value crop cotton. Barley and wheat are dry season crops, while maize, sorghum and cotton are wet season crops. Rice is grown in both seasons. Historical agricultural data was acquired from ICRISAT (http://vdsa.icrisat.ac.in/) and historical data of temperature and precipitation were acquired from APHRODITE (http://chikyu.ac.jp/precip/) (see S1 and S2 for further details).
The empirical model of irrigation decisions assumes that the planting decision has already been made. Therefore, irrigation decisions reflect the second stage in a farmer's decision making process, and each crop regression only accounts for the sample of districts that grow a particular crop over our study period, 1970–2005. We estimate the following equation for each crop:
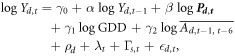
where is the irrigated area of a particular crop in district d in year t;
is a lag of the dependent variable, which captures spillover effects from investments in irrigation infrastructure affecting all subsequent irrigation decisions;
which follows from previous research [15, 18], is a vector of precipitation measures that represents June–September monsoon rainfall and the number of days with precipitation >0.1 mm;
is the previous 5 year average crop area, which captures the expectation to plant in the current period and growing degree day (GDD) captures GDDs by season calculated by using daily mean temperature [19].
In this model, we use short-run random variation in climate in a given area to compare that area's outcomes under different weather conditions after controlling for observed and unobserved characteristics using regional fixed effects, and a time fixed effect that further neutralizes any common trends. These time fixed effects are represented by
and
accounts for all common contemporaneous trends, for example, national price changes, economic growth and population growth while state-specific trends,
allow to control for differential trends by state such as state-wise technological progress and changes in electricity subsidies. In this way, a district observed during a dry year, acts as a 'control' for that same district observed during a wetter 'treatment' year. This is important because, for instance, one district might be wetter than another district, and also much wealthier. If irrigation decisions are a function of wealth, then a statistical model that just compares precipitation to irrigation outcomes between the two regions, without accounting for income differences, is likely to be biased. A similar modeling approach has been used in other studies that estimate agriculture–weather relationships in India [15, 18, 20–22], and in other countries [23, 24].
We adopt linear and Tobit regression approaches when modeling irrigated area. Zero irrigated areas reflect optimal outcomes of a decision and are modeled using a Tobit approach. Standard errors are clustered at the district level in the Tobit models, and corrected for spatial and serial correlation in the linear models [25].
2.2. Irrigated area projections
We combine our estimated historical irrigation response to weather (table 1) with bias-corrected climate projections from 5 general circulation models (GCMs) that have contributed to the World Climate Research program's Coupled Model Inter-comparison Project phase 5 (CMIP5) under the RCP 8.5 scenario [26]. The 5 GCMs used in this study are CCSM4, GFDL-CM3, GFDL-ESM2G, MIROC-ESM-CHEM, and NorESM1-M (table S1). We chose these models because they (i) come closest to characterizing India's historical monsoon [27, 28], and (ii) demonstrate a wide distribution of future climate changes, including both increases and decreases in monsoon rainfall and the number of rainy days within the monsoon season (figure S3).The bias correction method and number of models used follows the Inter-Sectoral Impact Model Inter-comparison Project approach [29]. Further details are in sections S2 and S3.
Table 1. Regression estimates on weather-related variables in agro-economic model.
Wet season | Dry season | ||||||
---|---|---|---|---|---|---|---|
(1) | (2) | (3) | (4) | (5) | (6) | (7) | |
Rice | Maize | Sorghum | Cotton | Rice | Wheat | Barley | |
No. of rain days | 0.03 | −0.327*** | −0.054+ | −0.199*** | −0.003 | −0.005 | −0.180* |
(0.038) | (0.087) | (0.030) | (0.000) | (0.032) | (0.071) | (0.091) | |
Rainfall JJAS | 0.070*** | −0.014 | −0.039*** | 0.045*** | 0.166*** | 0.250*** | −0.026 |
(0.020) | (0.019) | (0.011) | (0.000) | (0.023) | (0.034) | (0.043) | |
Wet GDD | −0.048 | −0.099* | 0.027 | −0.310*** | 0.301** | 0.089 | 0.066 |
(0.040) | (0.039) | (0.027) | (0.000) | (0.115) | (0.069) | (0.110) | |
Dry GDD | −0.316 | −0.159 | 0.068 | ||||
(0.243) | (0.099) | (0.184) | |||||
Model | Tobit | Tobit | Tobit | Tobit | Tobit | OLS | OLS |
N | 8248 | 7244 | 5178 | 3244 | 3770 | 7460 | 3882 |
0 Observations | 632 | 1628 | 3520 | 277 | 586 | 19 | 128 |
Average partial effects for Tobit models and coefficient estimates for ordinary least square (OLS) models represent the effect of monsoon precipitation (June–September), rainfall distribution (number of rainy days in June–September), and seasonal GDD on irrigated area decisions, 1970–2005. A Tobit model is used for crops where a large fraction of the observations are clustered at 0. Standard errors in parentheses are clustered at the district level for the Tobit models and corrected for spatial and serial correlation for OLS models. The dependent variable is the logarithm of district-level crop irrigated area in 1000 ha. All regressions include district and year fixed effects, and state-specific trends to control for time-invariant district characteristics, country-wide trends, and time-varying differences between states. See S1–S4 for data sources, results for non-weather variables and robustness checks. Statistical significance is given by + p < 0.10 * p < 0.05 ** p < 0.01 ***p < 0.001.
2.3. Hydrology model: physical system
Projected changes in irrigated area and bias-corrected CMIP5 climate projections are inputs to the hydrology model, WBMplus [30, 31]. WBMplus simulates vertical water exchange between the land surface and the atmosphere, and horizontal water transport through runoff and stream networks [30], and computes irrigation water demand, supply, and use by crop type [31]. WBMplus is a gridded, process-based, hydrology model [30, 31], which here uses a 30 arcminute grid resolution with the simulated topological river network STN-30p [32, 33]. The climate inputs—both historical and future—are the same as used for the econometric analysis and projections (SI sections S2 and S3). WBMplus simulations were run for each GCM climate projection separately, including a 10 year spin-up with each GCM's historical output (1996–2005). Historical agricultural data (i.e., irrigated area by crop type) is from ICRISAT (SI section S1), and future agricultural data is from the econometric model projections. The field capacity and wilting point of soils is a required input for calculating both crop and non-crop potential evapotranspiration. We use the FAO/UNESCO Soil Map of the World [34] for both, as well as for the soil drainage classes. Soil drainage class is used to estimate water seepage through flooded rice paddies. Large reservoirs are represented as river segments with the reservoir's storage capacity; data on large reservoir capacity, location and primary purpose are from the GRanD database [35].
2.4. Unmet irrigation demand: modeling the loss of groundwater access
We quantify current (c.2000) irrigated crop production that can be attributed to UGW by simulating historical crop water requirements as described above, but restricting irrigation water supplies to sustainable sources. Unmet irrigation water demand was assumed to lead to contraction of irrigated areas [36]. We apply the contraction of irrigated area equally to all crops within a district. This is a first-order assumption; water deficits will likely cause uneven distribution of irrigated area contractions due to a range of factors. These factors may include the profitability of the crop type, the ability of the crop to survive under deficit irrigation conditions, or the crop water productivity of a crop type. Results shown here should be considered as estimates of crop production losses due to UGW restrictions; further work on this topic will help increase the accuracy of estimates shown here.
In the dry season, reduction of irrigated areas is assumed to equal a reduction in crop area, as most dry season crops cannot be grown without irrigation. In the wet season, we assumed farmers would grow the same area of crops, but under rainfed conditions. Therefore, dry season crop production losses, Ploss,d (tons), due to UGW restrictions are:

and wet season crop production losses, Ploss,w (tons), due to UGW restrictions are:

where Iunmet,d(w) is dry (wet) season unmet irrigation water demand, Igross,d(w) is the dry (wet) season gross irrigation water demand AN is the area (ha) of crop N, and IYN (tons ha−1) is the irrigated yield of crop N, and RYN (tons ha−1) is the rainfed yield of crop N, and Igross,w (km3) is the gross irrigation demand in the wet season. Crop production data is from ICRISAT (http://vdsa.icrisat.ac.in/).
2.5. National river linking project
To assess the NRLP's potential to reduce India's groundwater stress, WBMplus modeled all published proposed irrigation water transfers across river basins [37]. Two scenarios were simulated: one implements only the inter-basin water transfers through the proposed canal system; the other additionally adds reservoirs at each water recipient location. In both simulations, the daily volume of water moved through the canals is a function of river discharge at the donor location and the canal's capacity. In the second simulation, reservoir capacity is added to allow water transferred during the wet season to be stored until it is needed for irrigation in the dry season. We recognize that this simulation is highly speculative, as few details of the proposed increase in water storage have been published. Further details are in section S5.
2.6. Method limitations
The methods described above allow a human decision-making process—i.e., expansion or contraction of crop-specific irrigated areas—to be fully integrated into a physical hydrology system analysis. While inclusion of the human decision-making process is an important advance, the methods used here have several key assumptions which lead to several limitations. First, projections of irrigated areas assume that current trends in variables other than weather continue into the future: e.g., population growth, GDP growth, and technological advancement are all assumed to continue along their current trajectory. Therefore, this model system cannot account for significant extreme events or shocks to the economic or technological system. Second, projections of irrigated areas can only be made in districts that had crops planted in the historical assessment period. This limits the study by not allowing us to project the expansion of agriculture (irrigated or rainfed) into new areas. Third, the hydrologic model simulates crops that are either fully rainfed, or fully irrigated—deficit irrigation, the practice of providing only a portion of the amount of irrigation water required for optimal growth, is not simulated. Crop yields are not perfectly linear with added water and the relationship between water additions and crop yields vary between different crops [15, 18]. Therefore, when modeling the crop yield loss due to losing access to UGW, our methods only provide a first-order estimate. Fourth, groundwater levels drop unevenly across the country, and so loss of access to UGW will occur at different times in different places. The methods presented here do not capture this temporal variability, nor do they capture the potential human response to a gradual loss of UGW. Lastly, the simulation of the NRLP is based on imperfect knowledge of the location and storage capacity of planned reservoirs or other water storage systems [38]. The simulation results shown here are meant to optimize water storage and release volumes and timing, and therefore may not be representative of actual reservoir construction or storage volumes.
The methods used here and their limitations define the scope of this study: we are able to (a) project how changes in climate (and continued trends in all other relevant variables) will lead to human-based changes in crop-specific irrigated areas, (b) quantify how much irrigation water these projected irrigated areas will require under the same set of climate change conditions, (c) quantify how much UGW would be required to fully meet these projected irrigation water requirements, (d) categorize changes in the rate at which groundwater levels will drop in response to these projections, (e) estimate the quantity of food produced as a direct result of current UGW use and (f) estimate a potential range for UGW alleviation under full implementation of the NRLP.
3. Results
3.1. Monsoon impact on historical irrigated areas
The first step in assessing the future of irrigation in India is to quantify how historical changes in climate have driven historical patterns of irrigation. This understanding allows us to project changes in irrigation into the future, based on a suite of potential climate change scenarios. India has a monsoonal climate with a wet (Kharif) season that receives up to 1 m of rainfall and a dry (Rabi) season in which precipitation is insufficient to grow most crops and irrigation must be used. Consequently, farmers assess the supply of rain during the monsoon season and the amount that gets stored at the end of the season, in order to make decisions about increasing or decreasing irrigated areas for different crops [15]. Thus, there is a significant link between monsoon rainfall and irrigated areas in India [36, 39]. The future of monsoon rainfall is extremely uncertain; some climate change studies show an increase in future precipitation [40], others predict a decrease [41] (figure S3). Increases in inter-annual and intra-seasonal variation are also expected [27] along with rising temperatures [40] (figure S4). Such climatic change will affect irrigation water demand and supply due to farmer irrigation decisions, water supply, and physiological crop water requirements.
The econometric model estimates the effect of total precipitation, rainfall distribution, and seasonal GDD on seasonal, crop-wise irrigation decisions for six major crops in India, which make up 80% of India's crop production (table 1), across districts in all major agricultural states in India from 1970 to 2005. The logarithmic specification of the model enables interpretation of coefficients as elasticities, so that a 1% increase in the weather variable affects irrigated area by where
is the coefficient of interest. Precipitation and GDD have less than proportionate impacts on irrigation decisions (i.e.,
).
Precipitation plays a larger role than GDD in driving changes in irrigated area (table 1). The number of rainy days (i.e., the distribution of monsoon season rainfall) directly affects wet season crop irrigation, as too many days without rain during critical crop stages can reduce yields or lead to crop failure [42]. Supplemental irrigation in the wet season, largely relying on stored monsoon rainwater from previous years, can help overcome this uneven distribution of rainfall, but may not be able to offset decreases in total precipitation. Negative coefficients on the number of rainy days for maize (p < 0.001), sorghum (p < 0.1) and cotton (p < 0.001) reflect a rise in irrigated areas for most wet season crops in response to fewer rainy days, even when total rainfall is controlled for. In contrast, the impact of the total amount of rainfall on wet season irrigated area of rice, sorghum and cotton is varied. Of the three crops, sorghum is least water intensive and most drought resistant [43], so a fall in monsoon rainfall can be easily compensated by supplemental irrigation to meet its irrigation needs. Rice and cotton are more water intensive, rice due to the practice of flooding paddy fields, and cotton due to high crop water requirements for optimal growth [43]. As a long duration crop, cotton sometimes extends into the dry season, increasing its water needs substantially. For these two crops, coefficients on the total amount of rainfall are positive and significant (p < 0.001), implying that a reduction in total monsoon rainfall also decreases irrigated area. In the irrigation-intensive dry season, the capacity to irrigate rests on the amount of monsoon rainfall collected in surface and groundwater storage. Any decrease in precipitation during the previous monsoon season significantly (p < 0.001) reduces the area of rice and wheat that are irrigated. Barley, another dry season crop, is of short duration, hardier than wheat, relatively drought resistant [43] and relies on conserved soil moisture for its water needs [44]. A more even distribution of monsoon rainfall helps retain soil moisture and can significantly (p < 0.05) decrease barley irrigation.
The impact of GDD on irrigation is limited, with higher wet season GDD significantly contracting irrigated area for only maize (p < 0.05) and cotton (p < 0.001). Studies suggest that with an increase in temperature and water stress on plants, farmers tend to contract agricultural activity to smaller areas during the season [39]. Higher wet season GDD can also affect irrigation in the ensuing dry season, but in the opposite manner. We find that dry rice irrigation significantly (p < 0.01) increases in response to a rise in wet season GDD.
The crop-specific understanding of links between climate and irrigation presented here are necessary to generate projections of irrigated areas for each crop individually, since they are key to understanding future water requirements, as crops have varying levels of water requirements.
3.2. Projections of future irrigated areas under climate change
Farmers in India tend to respond to water scarcity along the extensive margin, changing the extent of cultivated and irrigated area rather than the rate of water use per unit area [36]. We combine our elasticity estimates (table 1) with predicted changes in precipitation and GDD from five different GCMs to project crop-specific irrigated areas (figure 2). The GCMs used model the historical Indian monsoon well [27, 28], yet they also span the range of positive to negative projected changes in future monsoon rainfall (figure S3). These irrigated area projections implicitly assume that historical irrigation decisions in response to changes in precipitation and temperature continue into the future, and that any future adaptation to a changing climate is fully embodied in the observed ability to adapt to past changes.
Figure 2. Econometric model-projected aggregate dry season (red) and wet season (blue) irrigated areas. Historical period (1970–2005) data is from ICRISAT. Future period (2006–2050) solid line is the multi-model mean of projections based on 5 GCM climate futures, with a shaded range of uncertainty due to GCM differences.
Download figure:
Standard image High-resolution imageIn developing countries like India, the majority of farmers face credit constraints, incomplete markets, lack of information, and low levels of human capital, which limits their ability to quickly adopt new technologies or improve upon existing ones [45, 46]. Thus, the estimates from our panel data models reflect the effects of climate change in the short- to medium-term scenarios, where farmers might be unable to adjust or re-optimize their decisions, or can only do so very slowly [45]. Recent research suggests that the degree to which people adapt to longer-run changes in temperature and precipitation reflects surprisingly little adaptation [47]. Thus, our econometric model evaluates the effects of climate on changes in irrigated area in the medium term (up to 2050), while keeping cropping decisions, growing seasons, and other variables unchanged and assuming trends in technology and population stay constant into the future.
Since the application of irrigation to cultivated cropland is a short-term adaptation response by farmers in the face of inter-annual monsoon variation, irrigated area projections are made year to year and we convert the estimated % changes in irrigated area into absolute values using the previous year's irrigated area as the base. While we acknowledge that the path of development in India will change in the future, it is nevertheless instructive to project irrigated areas to assess the possible magnitude of climate-change related UGW needs. We project irrigated area increases in both seasons, with uncertainty (±15% in the wet season, ±50% in the dry season by 2050) due to the range of GCM-projected future climates (figure 2). These increases are due to the extent of irrigated wheat and rice continuing their historical rising trend, while irrigated extent of other crops remains the same or decreases (figure S5).
3.3. Impacts of climate change on UGW abstraction
UGW abstraction is unsustainable in the long term, and can exhaust groundwater resources if continued unabated [7]. We use a process-based hydrology model that separately models both sustainable irrigation—that supplied by groundwater recharge and surface water rivers and reservoirs—and UGW. By integrating the agro-economic econometric model with the hydrology model, we assess the impact of climate change and the resulting changes in irrigated areas on future UGW demand (figure 3)
Figure 3. Trends in district-level ground water levels (GWL) between 1979–2000 and 2029–2050, inferred from the multi-model mean of changing need for unsustainable groundwater (UGW) to meet irrigation water needs. Decreases in UGW demand will slow down GWL declines (yellow); continued demand will lead to continued GWL declines (red); increased demand will increase GWL declines (dark red); new positive demands can start GWL declines (orange); demand going to 0 can allow GWL to recover (blue). Black lines are state boundaries. Colored (non-gray) regions account for 90% of future modeled national UGW demand. Figure S6 shows trends in GWL for 5 individual GCM climate futures.
Download figure:
Standard image High-resolution imageIndia's northwest region has already experienced significant groundwater level decreases due to UGW use [6]. We use our model projections of future UGW demand to infer how groundwater levels will change up to 2050. If demand increases, then groundwater levels will drop more rapidly (figure 3, dark red); continued demand will lead to continued rates of groundwater level decline (figure 3, red), while reduced but positive demands will slow the rate of groundwater level decline (figure 3, yellow). Some districts will be able to rely solely on sustainable water supplies, allowing groundwater levels to recover (figure 3, blue). Under future climate change, most of Punjab and Haryana, northern areas of Rajasthan and Gujarat and parts of Uttar Pradesh and Tamil Nadu will face continued and further groundwater level declines (figure 3). Additionally, the spatial extent of UGW pumping expands to pockets of Tamil Nadu, Andhra Pradesh, Uttar Pradesh and Gujarat, regions that previously did not over-abstract groundwater (figure 3, orange).
Free or flatly tariffed electricity provisions have played a critical role in enabling groundwater extraction [48] and might further contribute to UGW use if present day irrigation and cropping practices persist. However, despite the presence of subsidies, expensive pump technology is still needed to draw groundwater once levels drop beyond certain thresholds [49]. Therefore, evidence of continued and increased future groundwater level declines reflect potential constraints on access as rising pumping costs can eventually make extraction prohibitive.
To assess how a loss of access to UGW may affect Indian agriculture, we quantify the amount of unmet irrigation water demand that will occur in its absence by restricting the use of the UGW within the hydrology model (figure S7). Without UGW, unmet irrigation water demand will reach 170 km3 yr−1 by 2050 (figure S7), paralleling only the unmet demand in 2002, a year in which India was hit by a massive drought [50].
3.4. Policy implications
Losing access to UGW directly translates to reductions in food production. We find that currently, half of dry season irrigated crop production and a quarter of the total annual irrigated crop production is directly sustained by UGW (table 2). The most affected regions primarily grow India's staple crops—wheat and rice—in the dry season [51]. The fertile Indo-Gangetic Plain is one of the most intensely farmed and populated areas in the world, and includes much of Uttar Pradesh, Punjab and Haryana, which have districts that produce up to 1.8 million tons of UGW-based agricultural output each year (figure 4). The southeastern states of Tamil Nadu and Andhra Pradesh also rely heavily on UGW for crop production, with some districts producing up to 0.8 million tons yr−1 using UGW (figure 4).
Table 2. The impact of unsustainable groundwater (UGW) on irrigated agriculture and food supply.
Irrigated agriculture production (million tons) | Production loss in absence of UGW (million tons), (% of total production) | Calorie loss in absence of UGW (billion kcal] | # People fed by UGW-dependent caloriesa (millions) | |
---|---|---|---|---|
Dry season | 75.4 | 38.7 (51%) | 121 300 | 166 |
Wet season | 73.4 | 2.8 (4%) | 4750 | 7 |
Annual total | 148.8 | 41.5 (28%) | 126 000 | 173 |
Figure 4. District-level reduction in current (c. year 2000) annual crop production, in million metric tons, that would occur if unsustainable groundwater supplies became unavailable. Black lines are state boundaries.
Download figure:
Standard image High-resolution imageTherefore, in the event that UGW becomes difficult to access, national food security will be threatened. We find that UGW in India is directly responsible for production of sufficient food calories to feed 173 million people, accounting for 14% of India's population (table 2). In a country where ∼194 million people go hungry every day [52], losing access to UGW would further aggravate food security concerns that already plague India.
Based on the range of projected future climates, UGW will either remain at historical levels (30–40 km3 yr−1), or it will increase to as much as 170 km3 yr−1 (figure S7). Historically, the irrigation water deficit that would occur due to restricting UGW use was 5%–15%. Future irrigation water deficits may stay at this level, or increase up to 40%. If the future UGW demand remains the same as the present, then the food cost of eliminating UGW use will remain at levels summarized in table 2. However, the extreme case of increasing irrigation water deficits to 40% would result in significantly larger reductions in food production. However, it is worth noting that future advances in agricultural systems may allow for higher crop yields under water stressed conditions. Additionally, increased irrigation efficiency may allow for more water to be used beneficially by crops even with reduced water extractions.
A recent government initiative has looked to the massive NRLP to overhaul water management in India. The $123 billion project intends to move 178 km3 yr−1 of water by connecting 37 rivers, building ∼3000 storage dams and 12 500 km of water conveyance networks [17, 38]. If completed, it will be the biggest infrastructure project in the world [38]. In addition to its stated goals of 34 GW of hydropower generation, increasing drinking water supplies, and mitigating floods in the east [38], it is also expected to alleviate the stress on groundwater. The NRLP is expected to increase the extent of irrigated agriculture by 35 million ha through surface irrigation and improved groundwater recharge [38].
To quantify the NRLP's impact on UGW demand and surface water irrigation, we simulate a scenario in which all proposed river links are functional along with concurrent construction of large reservoirs at receiving nodes in the NRLP, and compare the UGW demand to our baseline model results. We find that with both the additional reservoirs and the inter-basin transfer network functioning, there is potential to alleviate 10%–16% of India's mid-century UGW demand (figure 5(b)). However, without new large reservoirs, the inter-basin transfers alone reduce only 1%–4% of overall UGW demand (figure 5(c)). Historically, construction of large dams has been contentious in India [53]. While the exact plans for dam construction under the NRLP have not yet been publicized, it is clear from these results that without a large increase in reservoir capacity, the NRLP will not alleviate groundwater stress in northwest India.
Figure 5. (A) Mid-century annual unsustainable groundwater (UGW) demand at the district level. The national river linking project (NRLP) is a proposed solution for alleviating this demand. (B) and (C) Light blue lines: NRLP water transfer canals; red dots: water donor locations; blue dots: water recipient locations. Blue dots along chained canals are both receiving and donating. (B) The % of each district's mid-century UGW demand that could be alleviated with the implementation of NRLP canals and construction of new reservoirs along canal routes. Blue: UGW demand is alleviated; yellow and red: UGW demand is worsened. National total UGW alleviation is 10–16%. (C) The % of each district's mid-century UGW demand that could be alleviated with the implementation of NRLP canals only. National UGW alleviation is 1%–4% with transfers only. Gray lines are state boundaries. (B) and (C) share a scale bar.
Download figure:
Standard image High-resolution imageThe NRLP is expected to cost $123 billion USD; it aims to both expand irrigated areas ($64 billion) and grow the country's hydropower capacity ($59 billion) [54], both of which are expected to provide economic returns on this investment. However, it is worth comparing this cost to the value of current groundwater agricultural production and the cost of past irrigation projects. Irrigation by groundwater—both sustainable and unsustainable—currently (circa year 2000) contributes 7.6–8.3 billion USD per year to India's agricultural output, which is slightly (∼8%–10%) more than the contribution of surface water irrigation [55]. Since 1990, the government has invested more than $ 24 billion USD in surface irrigation but little increases in net surface irrigated area have been realized [54] Instead, the share of groundwater-based versus surface water-based agricultural output has been growing over the past 30 years [55], reflecting the increased reliance of the agricultural sector on groundwater resources. Protecting this sector from the loss of a large portion of water resources in the future may reflect a value of more than 7.6–8.3 billion USD per year; further analysis of the impact of replacing groundwater irrigation with NRLP-enabled surface water irrigation is needed for a full cost-benefit analysis. India's past irrigation projects have had a wide range of investment costs, from 190 USD/ha to 4560 USD/ha [56]. If the NRLP succeeds in expanding irrigated areas by 35 million hectares [38], it will cost 1829 USD/ha. While this cost is within the range of past irrigation projects, the expected irrigated area expansion does not account for the need to switch currently groundwater irrigated areas to surface water irrigated areas. Failure of previously-planned large irrigation projects has led to concern over the cost of the NRLP [56], while analysis of non-failed large-scale irrigation projects has shown that there is no difference in the average economic performance between successful large-scale and medium- to small-scale irrigation projects and that the management of irrigation projects is more important than their scale [56].
Alternative methods of reducing UGW demand may have lower costs and should be compared to the the NRLP. Examples of such measures include increasing irrigation efficiency, improving the timing of canal water deliveries to irrigated fields, and growing less water-intensive crops during the dry season. Assessing the cost of each of these alternatives is not within the scope of this paper; future work on this subject should compare costs of alternative water saving methods to the NRLP cost reported here.
4. Discussion
In this paper, we project future UGW use by accounting for changes in both demand and supply of water and their interaction under different climate change scenarios. We are able to identify regions where groundwater demand and supply may change significantly (figures 3 and 4), which has important implications for policy decisions that affect agricultural development, poverty, food security and adaptation.
One policy recommendation for managing water is the NRLP, but our results demonstrate canals alone have limited ability to decrease unsustainable use of groundwater nationally (figure 5(c)). In addition to the NRLP, several other policy and infrastructure tools have been studied, and research has indicated they have potential for reducing groundwater abstraction in different areas. Interventions researched include investments in public provision of groundwater to crowd out private construction of wells [49], decentralized rainwater harvesting schemes [8], creation of groundwater markets [57], metering electricity [58], and power supply rationing by separating agricultural from non-agricultural feeders [59]. Moreover, our conceptual framework illustrates additional adaptation measures and competing demands from other sectors that could potentially impact water supplies in the face of climate change (figure 1). Further assessment that accounts for these additional components in conjunction with those studied in this paper can help to identify potential synergies and conflicts, and remains an important area of future work. In the end, our coupled model suggests that, regardless of the mechanism, support for sustainable groundwater management will become increasingly urgent in the near future.
5. Conclusion
The results of this study provide the first multidisciplinary assessment of the extent of UGW use in India through mid-century, its importance in sustaining food production, and the potential role of large infrastructure projects in decreasing India's dependence on UGW. Our results emphasize that under a business as usual scenario, climate change induced precipitation increases in certain areas will not necessarily alleviate groundwater stress, due to the expansion of irrigated areas. This analysis also points to the need for a thorough analysis of farmer decision-making responses to infrastructure projects such as the NRLP and other policy measures that affect the availability of irrigation water supplies, as it is likely that subsistence-level food security concerns may drive these decisions. While we quantify here the potential for the NRLP to alleviate groundwater stress, it is possible that expansion of irrigated areas in response to the project (as has been promoted by NRLP-planners) will negate these potential benefits.
Competing financial interests
The authors declare no competing financial interests.
Acknowledgments
This work was supported by the National Science Foundation, Water Sustainability and Climate program (EAR-1038614), the US Department of Energy, Office of Science, Biological and Environmental Research Program, Integrated Assessment Program (DE-SC0005171), the National Science Foundation Graduate Research Fellowship Program (DGE-0913620), and by a grant from the National Science Foundation's Sustainable Research Network program (cooperative agreement GEO-1240507). Details about data reported in the paper is provided in the SI. We acknowledge the World Climate Research Programme's Working Group on Coupled Modeling, which is responsible for CMIP, and we thank the climate modeling groups (SI appendix, table S1) for making their outputs available. For CMIP, the US Department of Energy's Program for Climate Model Diagnosis and Intercomparison provides coordinating support in partnership with the Global Organization for Earth System Science Portals.
Author contributions
EZ and DSG contributed equally to this work; EZ, DSG, KFV, SF, RBL, and DHW designed research; EZ, DSG, KFV, SF, RBL, DHW, and AP performed research; EZ, DSG, KFV, SF, RBL, DHW and REN analyzed data; and EZ, DSG, KFV, SF, RBL and DHW wrote the paper.